A Deep Transfer Learning Solution for Food
Material Recognition using Electronic Scales
Tree Adaptation Network (TAN)
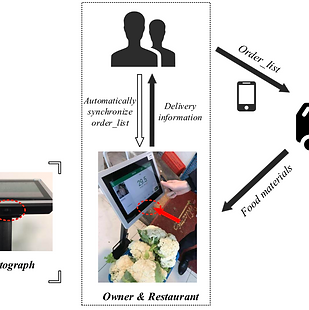
Abstract
We present a novel solution to automating procurement of food materials using electronic scales, which can automatically identify the food materials along weighing them. Though CNN model is regarded as one of the most effective solutions to image recognition, however, the traditional techniques cannot handle the mismatch problem between the lab training data and the real world data. To solve the problem, we propose to embed a partial-and-imbalanced domain adaptation technique (Tree Adaptation Network) in the deep learning model, which can
borrow knowledge from sibling classes, to overcome the imbalance problem, and transfer knowledge from the source domain to the target domain, to fight the mismatch problem between the lab training data and the real world data. Experiments show that the proposed approach outperforms the state-of-the-art algorithms. Furthermore, the proposed techniques have already been used in practice.
Datasets
Download Meal300
The evaluation is conducted on two public available datasets: Office-31 and Meal-300.
Office-31 [38] is a well known deep transfer learning dataset in computer vision, which includes 31 classes with 4652 images for three domains such as Amazon, Webcam, and DSLR.
Meal-300 dataset is collected, cleaned, and annotated from the electronic scales system, which is a food material supply chain system and serves about 1,000 restaurants, with most of them located in Hunan province of China. We annotated 53,374 clean images of 300 categories for the source lab domain. Fig. 5(a) shows the number of images for each category in the lab domain, which include 300 categories of food materials, ranging from 10 to 453, the data is significantly imbalanced, and the distribution of the super-classes are summarized as in Fig. 6, while most of them are vegetable and
meat.